10 Ways To Implement AI Solutions in Manufacturing
- webymoneycom
- Dec 21, 2024
- 16 min read
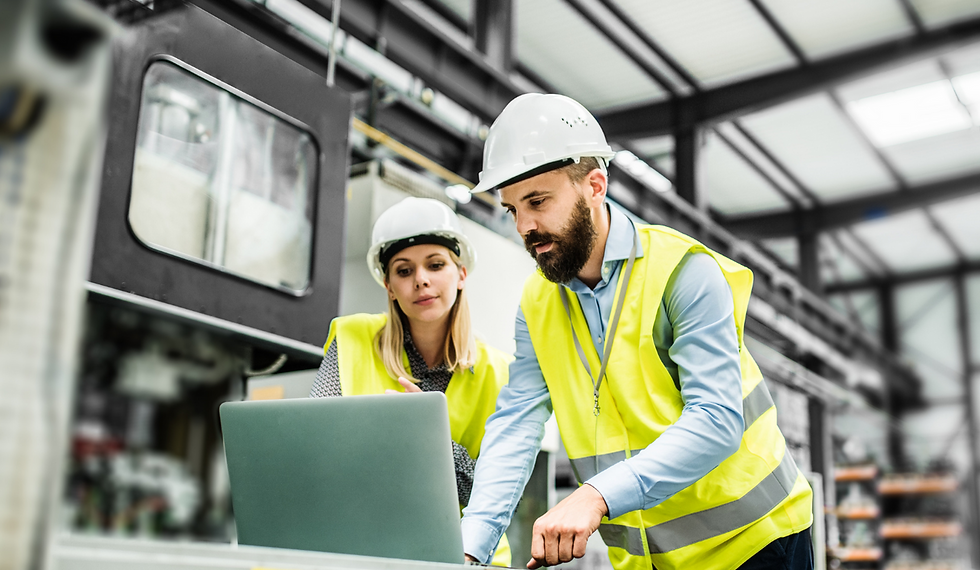
Would you like to transform your manufacturing processes?
In today's technology-driven world, remaining ahead of the curve is critical. That's where implementing AI solutions in manufacturing can make all the difference. From streamlining production to optimizing supply chain management, AI has the potential to take your operations to new heights. Picture this - predictive maintenance that minimizes downtime, quality control that exceeds expectations, and intelligent inventory management that maximizes efficiency. These are just a few examples of what AI can bring. The possibilities are truly endless.
But why stop there? With the power of AI, you can unlock insights from your data that were previously unimaginable. Imagine confidently making data-driven decisions, knowing you have a comprehensive and accurate understanding of your operations.
This blog will explore ten compelling ways to implement AI solutions in manufacturing. So, if you're ready to embrace the future of manufacturing and take your operations to the next level, you're in the right place. Get ready to be convinced of the transformative power of AI in manufacturing.
Let's dive in and unlock the potential together.
Implementing AI solutions in manufacturing can significantly improve efficiency, productivity, and quality. Here are ten ways to do so:
1. Predictive Maintenance

Predictive maintenance is a critical application of artificial intelligence (AI) in manufacturing, revolutionizing how companies manage their equipment and machinery. Traditional maintenance practices often rely on scheduled inspections or reactive repairs after a breakdown occurs, leading to costly downtime, unexpected production halts, and inefficient resource allocation. In contrast, predictive maintenance harnesses the power of AI algorithms and machine learning to analyze huge amounts of historical data, sensor readings, and operational parameters in real-time. By scrutinizing patterns and anomalies within this data, AI models can forecast potential equipment failures before they happen, enabling proactive maintenance interventions.
One of predictive maintenance's primary benefits is its ability to prevent unplanned downtime. By identifying early signs of equipment degradation or malfunction, manufacturers can schedule maintenance activities during planned downtimes or low-production periods, minimizing disruption to operations. This proactive approach not only improves overall equipment reliability and uptime but also extends the lifespan of machinery by managing problems before they escalate into significant failures. Consequently, companies can optimize their maintenance schedules, decrease maintenance costs, and improve the efficiency of their production processes.
Moreover, predictive maintenance enables a shift from conventional calendar-based or usage-based maintenance strategies to condition-based maintenance. Rather than replacing components on fixed schedules or after a certain number of operating hours, maintenance moves are triggered based on the actual condition and performance of the equipment. This approach maximizes resource utilization by ensuring maintenance activities are performed precisely when needed, avoiding unnecessary replacements or overhauls. As a result, manufacturers can minimize spare parts inventory, reduce labor costs, and achieve greater operational efficiency.
Furthermore, implementing predictive maintenance fosters a culture of data-driven decision-making within manufacturing organizations. Companies gain deeper insights into their assets' behavior and operational patterns by leveraging AI-powered analytics to monitor equipment health and performance continuously. This actionable intelligence enables them to fine-tune maintenance strategies, optimize asset performance, and identify opportunities for process improvement. Additionally, predictive maintenance systems can be integrated with enterprise asset management (EAM) or computerized maintenance management systems (CMMS), providing centralized oversight and control over organizational maintenance activities.
By leveraging AI to predict equipment failures, optimize maintenance schedules, and enhance operational efficiency, companies can minimize downtime, decrease costs, and improve overall productivity. As organizations in the manufacturing industry embrace digital transformation, predictive maintenance will continue to be a crucial technology. This will let them remain competitive in a dynamic and demanding market landscape.
2. Quality Control
Implementing computer vision systems powered by artificial intelligence (AI) for quality control is a transformative approach in modern manufacturing. Traditionally, quality control processes rely heavily on manual inspection, which is labor-intensive, subjective, and prone to human error. Manufacturers can automate and enhance the inspection process by leveraging AI-driven computer vision technology, ensuring consistent and accurate product quality assessment throughout the production line.
At the heart of AI-powered quality control systems lies computer vision algorithms capable of analyzing images or video streams in real time. These algorithms can quickly and accurately detect defects, anomalies, or deviations from quality standards. By training these algorithms on a diverse annotated image dataset, manufacturers can teach them to recognize various defects specific to their products or production processes. This training process, often facilitated by deep learning techniques, enables the AI system to continuously learn and improve its accuracy, adapting to evolving manufacturing requirements.
One key advantage of AI-driven quality control is its capability to detect defects that may be invisible to the human eye or easily overlooked by manual inspectors. Computer vision systems can inspect products at a microscopic level, identifying subtle defects, surface imperfections, or dimensional variations that may affect product performance or aesthetics. This level of granularity ensures that even the slightest deviations from quality standards are promptly identified and addressed before products leave the factory floor, reducing the likelihood of defective products reaching consumers and mitigating potential quality-related issues.
Moreover, AI-powered quality control systems offer unparalleled speed and scalability compared to manual inspection methods. With advancements in hardware acceleration and parallel processing, these systems can analyze vast quantities of visual data in real time, enabling high-speed inspection rates compatible with modern production line speeds. This accelerated inspection process improves throughput and productivity and facilitates early defect detection, minimizing rework, scrap, and rejections downstream in the manufacturing process.
Furthermore, AI-driven quality control systems provide valuable insights into production processes and product quality trends. By collecting and analyzing data from inspection results, manufacturers can gain actionable intelligence on factors influencing quality, such as machine settings, material variations, or environmental conditions. This data-driven approach empowers manufacturers to identify the root causes of defects, optimize production parameters, and continuously implement preventive measures to improve product quality and process efficiency.
3. Process Optimization
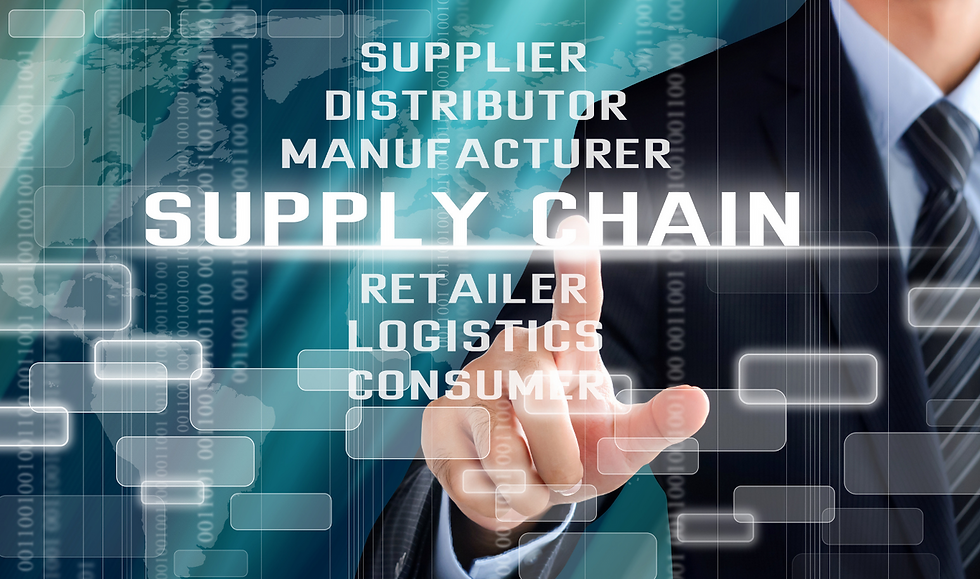
Implementing AI-driven process optimization holds immense potential for revolutionizing manufacturing operations by enhancing efficiency, productivity, and resource utilization. Traditionally, process optimization involves identifying inefficiencies, bottlenecks, and waste in manufacturing workflows and implementing corrective measures to improve overall performance. However, manual analysis of complex production processes can be time-consuming, subjective, and limited in its ability to uncover hidden patterns or correlations within extensive datasets. By contrast, AI algorithms can analyze large volumes of data from various sources, including sensor readings, production logs, and historical records, to identify optimization opportunities and recommend actionable real-time insights.
At the core of AI-driven process optimization is the application of machine learning algorithms capable of analyzing complex datasets to uncover hidden patterns, correlations, and trends. These algorithms can identify optimization opportunities across different aspects of the manufacturing process, such as production scheduling, resource allocation, inventory management, and workflow design. By continuously analyzing data streams and feedback loops, AI systems can adapt and refine their recommendations over time, ensuring that process optimization efforts remain aligned with changing operational requirements and market conditions.
One of the primary benefits of AI-driven process optimization is its ability to identify and mitigate bottlenecks in production workflows. AI algorithms can analyze real-time production data to identify manufacturing processes' congestion, inefficiency, or underutilization. AI algorithms can analyze real-time production data to identify manufacturing processes' congestion, inefficiency, or underutilization. This insight enables manufacturers to reconfigure workflows, allocate resources more effectively, and optimize production schedules to maximize throughput and minimize cycle times. By addressing bottlenecks proactively, companies can improve overall production capacity, reduce lead times, and enhance customer satisfaction.
Furthermore, AI-driven process optimization enables manufacturers to optimize resource utilization and minimize waste throughout the production lifecycle. AI systems can identify opportunities for resource conservation, recycling, and sustainable manufacturing practices by analyzing data on raw material consumption, energy usage, and waste generation. This reduces operational costs and supports environmental sustainability goals by minimizing the ecological footprint of manufacturing operations. Additionally, AI algorithms can optimize inventory levels by predicting demand fluctuations, supplier lead times, and production requirements, ensuring that companies maintain optimal stock levels while minimizing excess inventory holding costs.
Moreover, AI-driven process optimization facilitates continuous improvement by providing real-time feedback on production performance and quality metrics. By monitoring key performance indicators and analyzing production data, manufacturers can identify deviations from targets, root causes of inefficiencies, and opportunities for process refinement. This data-driven approach empowers companies to implement targeted interventions, iterate on process improvements, and drive ongoing optimization initiatives to enhance operational excellence and market competitiveness.
4. Supply Chain Management
Implementing AI in supply chain management introduces a paradigm shift in how manufacturing companies handle logistics, inventory management, and demand forecasting. Traditionally, supply chain management relies on historical data, manual forecasts, and predefined rules to plan and execute operations. However, modern supply chains' dynamic and interconnected nature necessitates a more adaptive and data-driven approach. By leveraging AI algorithms, manufacturers can analyze vast amounts of data from disparate sources, including sales records, market trends, supplier performance metrics, and external factors like weather patterns or geopolitical events, to gain real-time insights and optimize decision-making across the supply chain.
At the heart of AI-enabled supply chain management is applying advanced analytics techniques, such as machine learning and predictive modeling, to forecast demand accurately. By analyzing historical sales data, seasonality patterns, promotional events, and other relevant factors, AI algorithms can generate more accurate demand forecasts than traditional methods. This allows manufacturers to predict demand changes, optimize inventory levels, meet customer requirements, and minimize inventory holding costs and stockouts.
Furthermore, AI-driven supply chain management facilitates dynamic inventory optimization by continuously analyzing demand signals, supplier lead times, production capacity, and transportation constraints. By leveraging optimization algorithms, companies can determine the optimal inventory stocking policies, reorder points, and safety stock levels to balance the trade-off between inventory carrying costs and stockout risk. This adaptive approach enables manufacturers to respond quickly to changes in market demand, supply disruptions, or production delays while maintaining efficient inventory levels across the supply chain network.
Moreover, AI algorithms can enhance supply chain visibility and transparency by integrating data from various sources and providing real-time insights into inventory levels, order status, shipment tracking, and supplier performance. This end-to-end visibility enables manufacturers to identify potential bottlenecks, mitigate risks, and optimize decision-making throughout the supply chain. Supply chain management can be automated through AI to free up resources for strategic initiatives.
Furthermore, AI-driven supply chain management enables manufacturers to implement dynamic pricing strategies based on real-time market conditions, competitor pricing, and customer behavior analysis. Companies can utilize machine learning algorithms to optimize pricing decisions, respond quickly to changes in demand or competitive dynamics, and maximize profitability and market share. This data-driven pricing approach enables manufacturers to align pricing strategies and business objectives better while enhancing customer satisfaction and loyalty.
5. Autonomous Robots
Integrating autonomous robots powered by AI into manufacturing operations represents a significant advancement that can revolutionize various aspects of production processes. These robots are equipped with advanced sensors, computer vision systems, and AI algorithms that enable them to perceive their environment, make decisions, and execute tasks autonomously. By leveraging autonomous robots, manufacturers can enhance efficiency, flexibility, and safety in their operations while reducing reliance on manual labor and mitigating risks associated with repetitive or hazardous tasks.
One of the primary benefits of autonomous robots in manufacturing is their ability to improve productivity and throughput. Unlike human workers, robots can operate continuously without breaking or resting, enabling round-the-clock production cycles. Moreover, autonomous robots can perform repetitive tasks with high precision and consistency, improving quality and reducing manufacturing process variability. This increased productivity enables manufacturers to more effectively meet growing demand, scale production capacity, and achieve economies of scale.
Furthermore, autonomous robots enhance flexibility and adaptability in manufacturing operations. Autonomous robots are different from traditional fixed automation systems in that they can be easily reprogrammed or reconfigured to perform various tasks or adjust to changes in production requirements. This flexibility enables manufacturers to swiftly respond to shifts in market demand, product variations, or process improvements without incurring costly expenses for retooling or reengineering. Additionally, autonomous robots can collaborate seamlessly with human workers, augmenting their capabilities and enabling more efficient and ergonomic workflows.
Moreover, autonomous robots contribute to improved workplace safety by performing hazardous or physically demanding tasks that pose risks to human workers. By automating tasks like material handling, assembly, or welding in dangerous environments, robots help reduce the likelihood of workplace accidents, injuries, and occupational hazards. This enhances worker safety and minimizes downtime and liability costs associated with workplace incidents. Autonomous robots can be equipped with sensors and monitoring systems to detect and mitigate potential safety hazards in real-time.
Furthermore, autonomous robots enable manufacturers to achieve higher operational efficiency and cost-effectiveness. By optimizing material flow, reducing idle time, and minimizing energy consumption, robots help streamline production processes and reduce operational costs. Additionally, autonomous robots can be integrated with predictive maintenance systems to monitor their health and performance, enabling proactive maintenance interventions and extending their lifespan. This proactive approach reduces unplanned downtime, maintenance costs, and disruptions to production schedules, improving overall equipment effectiveness (OEE) and profitability.
6. Energy Management
Implementing AI-based energy management systems in manufacturing facilities offers significant potential to optimize energy consumption, reduce costs, and enhance sustainability. Traditional energy management approaches often rely on manual monitoring and static control strategies, which may not fully capture energy usage's dynamic and complex nature in industrial settings. By contrast, AI-based energy management systems leverage advanced analytics, machine learning algorithms, and real-time data to provide actionable insights and optimize energy efficiency across various processes and equipment.
One of the primary benefits of AI-based energy management systems is their ability to identify energy savings and optimization opportunities through data-driven analysis. By continuously monitoring energy consumption patterns, production schedules, and equipment performance, AI algorithms can identify inefficiencies, anomalies, and potential areas for improvement. This granular insight enables manufacturers to pinpoint specific sources of energy waste, such as equipment malfunctions, suboptimal settings, or process bottlenecks, and implement targeted interventions to optimize energy usage while maintaining production output.
Moreover, AI-based energy management systems enable predictive maintenance of energy-intensive equipment, such as motors, pumps, and HVAC systems. By analyzing historical performance data and sensor readings, AI algorithms can catch early signs of equipment degradation or impending failures, allowing maintenance teams to schedule preventive maintenance activities proactively. This proactive approach prevents breakdowns, decreases downtime, and extends equipment lifespan, leading to energy savings and operational efficiency.
Furthermore, AI-based energy management systems facilitate real-time optimization of energy usage based on dynamic factors such as production demand, weather conditions, and energy prices. By integrating with smart sensors, IoT devices, and energy management software, AI algorithms can adjust equipment settings, production schedules, and energy consumption patterns in response to changing conditions. For example, AI algorithms can optimize heating, ventilation, and air conditioning systems based on occupancy levels, outdoor temperature, and indoor comfort requirements, maximizing energy efficiency while ensuring occupant comfort.
AI-powered energy management systems enable manufacturers to participate in demand response programs and energy markets, improving cost savings and revenue opportunities. By leveraging predictive analytics and optimization algorithms, manufacturers can adjust their energy consumption patterns in real time to respond to peak demand events, grid constraints, or fluctuating energy prices. This flexibility allows companies to reduce their energy costs during high prices or earn revenue by providing grid services such as demand response or frequency regulation.
7. Demand Forecasting
Implementing AI algorithms for demand forecasting in manufacturing can significantly enhance operational efficiency, inventory management, and customer satisfaction. Traditionally, demand forecasting relies on historical sales data and manual analysis to predict future product demand. However, this approach often falls short of capturing complex demand patterns, seasonality, and market dynamics. By contrast, AI algorithms leverage advanced statistical techniques, machine learning models, and data analytics to analyze large volumes of historical sales data, market trends, and external factors to generate precise and timely demand forecasts.
One of the primary benefits of AI-based demand forecasting is its ability to capture and analyze complex patterns and correlations within the data. Through traditional forecasting techniques, AI algorithms can detect subtle trends, seasonal patterns, and demand drivers that may not be visible. Models can generate more accurate and reliable demand forecasts across different product categories, regions, and customer segments by considering multiple variables and data sources, such as sales history, marketing campaigns, economic indicators, and weather patterns.
Moreover, AI-based demand forecasting enables manufacturers to adapt quickly to changes in market conditions and buyer preferences. By continuously analyzing incoming data streams and feedback loops, AI algorithms can adjust real-time forecast models to reflect changing demand patterns, emerging trends, and unexpected events. This agility allows manufacturers to make informed decisions regarding production scheduling, inventory management, and resource allocation, ensuring optimal responsiveness to market demand fluctuations while minimizing stockouts or excess inventory holding costs.
Furthermore, AI-based demand forecasting facilitates better alignment between supply and demand, leading to improved inventory management and cost savings. Manufacturers can optimize inventory levels, reduce stockouts, and minimize excess carrying costs by generating more accurate demand forecasts. This optimization helps streamline the supply chain, improve cash flow, and enhance operational efficiency. Additionally, AI algorithms can identify opportunities for demand shaping and promotion optimization, enabling manufacturers to influence consumer behavior and maximize sales revenue while minimizing markdowns and inventory obsolescence.
Moreover, AI-based demand forecasting enables manufacturers to enhance customer satisfaction and loyalty by confirming product availability and timely delivery. By accurately predicting future demand, manufacturers can maintain optimal stock levels and fulfill customer orders promptly, reducing lead times and backorders. This improves order fulfillment rates, reduces order cycle times, and enhances customer experience.
Additionally, AI algorithms can analyze customer feedback, sentiment analysis, and social media data to identify emerging trends, preferences, and customer needs. This enables manufacturers to tailor their product offerings and marketing strategies to better meet consumer expectations.
8. Product Design and Prototyping
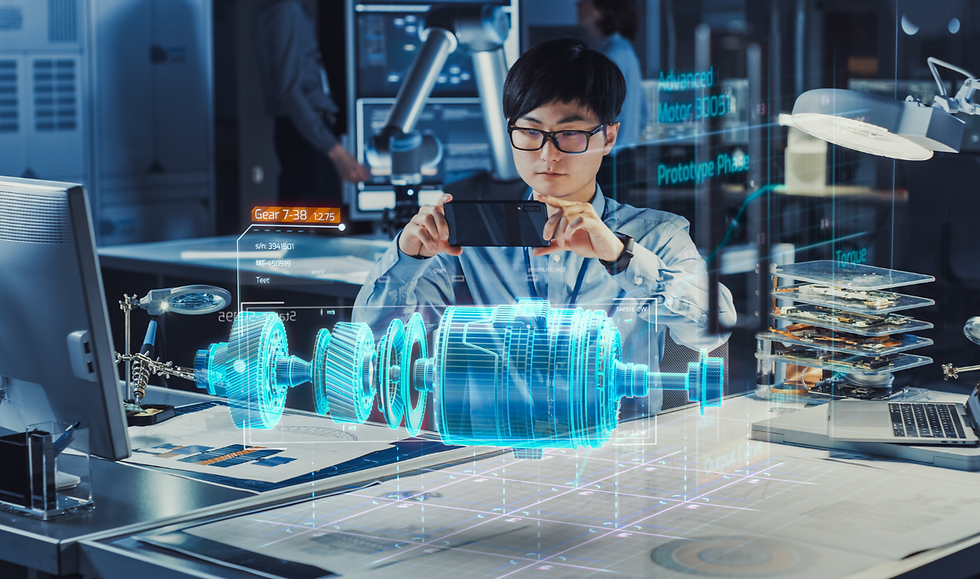
Implementing AI-driven design tools in manufacturing can revolutionize product development, leading to faster innovation, improved product quality, and enhanced competitiveness. Traditionally, product design involves iterative prototyping, testing, and refinement cycles, often requiring significant time, resources, and expertise. By leveraging AI-driven design tools, manufacturers can streamline and accelerate the design process, generate innovative designs, and optimize product performance while reducing costs and time-to-market.
One of the primary benefits of AI-driven design tools is their ability to generate and optimize product designs based on specified criteria and constraints. Using generative design algorithms and machine learning techniques, AI systems can explore vast design spaces, evaluate multiple design alternatives, and identify optimal solutions that meet specified performance requirements and design objectives. This enables manufacturers to discover novel design concepts, improve product functionality, and achieve better performance outcomes than traditional manual design methods.
Moreover, AI-driven design tools facilitate rapid prototyping and virtual testing, enabling manufacturers to validate and iterate on design concepts more efficiently. By simulating product performance under various operating conditions, AI algorithms can identify potential design flaws, structural weaknesses, or performance limitations early in the design process. This proactive approach reduces the need for physical prototypes, accelerates design iterations, and minimizes costly design changes downstream in the development cycle, ultimately leading to faster time-to-market and lower development costs.
Furthermore, AI-driven design tools enable manufacturers to continuously leverage data-driven insights and feedback loops to improve product designs. By analyzing data from simulation results, user feedback, and performance metrics, AI algorithms can identify areas for optimization, refine design parameters, and incorporate lessons learned into future iterations. This iterative design process fosters innovation, drives product improvements, and enhances overall product quality and competitiveness in the marketplace.
Designers and engineers can unleash their creativity by using AI-powered design tools to explore new possibilities. By augmenting human creativity with AI algorithms, manufacturers can overcome design constraints, explore unconventional design solutions, and unlock new opportunities for innovation. This collaborative approach lets designers focus on higher-level creative tasks, such as conceptualization and creativity. AI algorithms can handle repetitive or labor-intensive design tasks like optimization and iteration.
Moreover, AI-driven design tools facilitate collaboration and knowledge sharing across interdisciplinary teams and geographically distributed locations. AI systems facilitate seamless communication and collaboration between designers, engineers, and stakeholders by sharing design data, collaborating on design projects, and accessing shared repositories of design knowledge. This collaborative environment fosters cross-functional teamwork, accelerates decision-making, and ensures alignment between design objectives and business goals throughout the product development lifecycle.
9. Human-Machine Collaboration

Integrating AI systems and robotic technologies with human workers to enhance manufacturing productivity, efficiency, and safety is called human-machine collaboration. This approach recognizes the complementary strengths of humans and machines, leveraging AI to assist workers with complex tasks, provide real-time insights, and improve overall operational performance. By fostering collaboration between humans and machines, manufacturers can achieve greater flexibility, agility, and innovation in their production processes.
One of the primary benefits of human-machine collaboration is its ability to augment human capabilities with AI-driven automation and robotics. While humans excel at tasks requiring creativity, problem-solving, and adaptability, machines are well-suited for repetitive, physically demanding, or hazardous tasks. By deploying robots and AI systems alongside human workers, manufacturers can automate routine tasks, such as material handling, assembly, and inspection, while allowing humans to focus on higher-level cognitive tasks that require human intuition and judgment.
Moreover, human-machine collaboration enables manufacturers to achieve greater flexibility and adaptability in response to changing production requirements and market demands. By integrating AI-driven automation technologies with human workers, manufacturers can quickly reconfigure workflows, adjust production schedules, and accommodate variations in product mix or order volumes. This agility allows companies to respond more effectively to fluctuations in demand, supply chain disruptions, or changes in customer preferences, ensuring operational continuity and customer satisfaction.
Furthermore, human-machine collaboration enhances workplace safety by automating hazardous or physically demanding tasks and providing real-time monitoring and assistance to human workers. Robots with advanced sensors and AI can handle dangerous tasks while humans supervise from a safe distance. Additionally, AI systems can detect potential safety hazards, such as machine malfunctions or environmental risks, and alert human workers to take corrective actions, minimizing the risk of workplace accidents and injuries.
Moreover, human-machine collaboration fosters a culture of continuous learning and skill development among workers by providing opportunities for training, upskilling, and knowledge transfer. As AI technologies become increasingly integrated into manufacturing operations, workers can learn new skills, such as programming, robotics maintenance, and data analysis, in high demand in the digital age. Investing in human capital enhances employee engagement and job satisfaction and ensures workers remain competitive and adaptable in a rapidly evolving technological landscape.
Additionally, human-machine collaboration improves decision-making and problem-solving by providing real-time insights and analytics to support informed decision-making. AI systems can analyze data from sensors, production logs, and machine learning models to identify trends, anomalies, and opportunities for optimization. This actionable intelligence empowers workers and managers to make data-driven decisions, optimize production processes, and proactively address emerging challenges, improving operational efficiency and competitiveness.
10. Customer Insights and Personalization
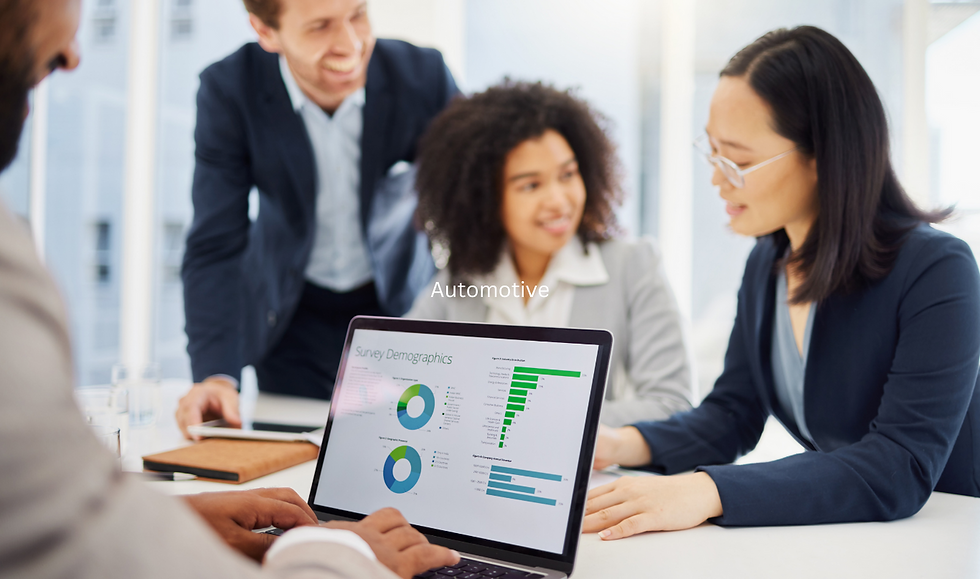
Implementing AI techniques like natural language processing (NLP) and machine learning (ML) in manufacturing facilitates the analysis of customer feedback, preferences, and behavior to enhance product offerings, marketing strategies, and customer experiences. Traditionally, manufacturers relied on market research surveys, focus groups, and sales data to understand customer needs and preferences. However, these methods often provide limited insights and may not capture the full spectrum of customer sentiments and expectations. By leveraging AI, manufacturers can analyze vast amounts of unstructured data from social media, online reviews, and customer service interactions to gain deeper insights into customer preferences, trends, and sentiment.
One of the benefits of utilizing AI for customer insights is its capability to process and analyze unstructured data at scale. Natural language processing (NLP) algorithms can extract useful information from textual data, like customer reviews, social media posts, and online forums, to determine patterns, themes, and sentiment analysis. By analyzing this unstructured data, manufacturers can uncover valuable insights into customer perceptions, preferences, and pain points. This enables them to tailor their product offerings, marketing messages, and customer service strategies to better meet customer needs and expectations.
Moreover, AI-powered customer insights enable manufacturers to personalize product offerings and marketing campaigns to individual customer preferences. Machine learning algorithms can analyze historical purchase data, browsing behavior, and demographic information to segment customers into distinct groups founded on their preferences, behaviors, and purchase patterns. By understanding each customer's unique preferences and purchasing behavior, manufacturers can personalize product recommendations, promotional offers, and marketing messages to enhance customer engagement and loyalty.
AI-powered customer insights help manufacturers anticipate market trends and preferences. By analyzing real-time data from social media, online forums, and other sources, manufacturers can identify emerging trends, sentiment shifts, and customer demands early in the product lifecycle. This approach lets companies adapt to market changes and outperform competitors quickly.
Additionally, AI-powered customer insights facilitate continuous improvement by providing feedback loops and actionable intelligence to inform product development and marketing strategies. By analyzing customer feedback, sentiment analysis, and product reviews, manufacturers can identify areas for product improvement, address customer concerns, and innovate based on customer preferences. This iterative process fosters innovation, drives product enhancements, and strengthens customer relationships, increasing customer satisfaction and loyalty.
Moreover, AI-driven customer insights enable manufacturers to optimize their sales and marketing efforts by identifying high-value customers, predicting purchasing behavior, and optimizing marketing channels. Machine learning algorithms can examine historical sales data, customer interactions, and demographic information to identify potential leads, segment customers, and personalize marketing campaigns for maximum impact. This data-driven approach improves marketing ROI, enhances customer acquisition and retention, and drives revenue growth for manufacturers in an increasingly competitive marketplace.
By leveraging AI in these areas, manufacturers can unlock new efficiency, agility, and innovation levels in their operations, ultimately driving competitive advantage and business growth.
The Bottom Line
AI solutions in manufacturing can revolutionize the industry by improving efficiency, productivity, and quality. Manufacturers can remain competitive in a rapidly evolving market by embracing AI-powered technologies such as predictive maintenance, quality control, and supply chain optimization. Companies must stay informed about AI advancements and carefully strategize implementation to reap the full benefits. With the right approach, AI can transform the manufacturing landscape, driving innovation and shaping the industry's future.
Comments