A Comprehensive Guide To Use Generative AI
- webymoneycom
- 4 days ago
- 16 min read
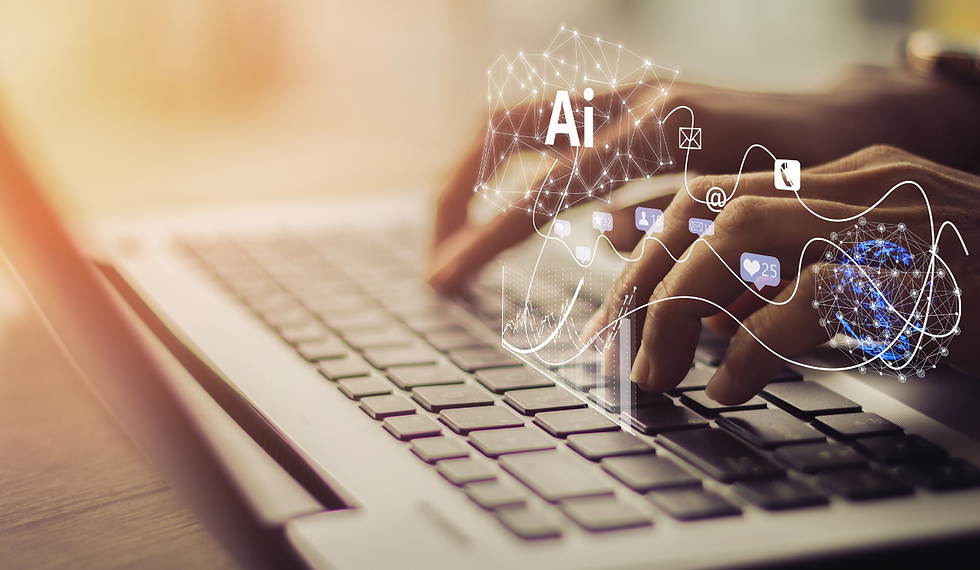
ChatGPT revolutionized the entire artificial intelligence industry when it burst onto the scene last year. This powerful AI tool can generate responses to nearly any inquiry it receives. Generative AI has the potential to replace long hours spent with a notepad and pen, and it's already being hailed as one of the most exceptional chatbots ever developed.
Generative AI represents the latest iteration of artificial intelligence that's grabbing headlines. Although this technology isn't entirely new, its ability to create genuine content suitable for marketing purposes is groundbreaking.
In this guide, we will discover the possibilities and applications of generative AI, highlighting how it can revolutionize various industries and creative endeavors.
So, get ready to embark on an exhilarating exploration of Generative AI. Whether you're an aspiring AI enthusiast or a seasoned professional, this guide is your ultimate companion to unraveling its potential.
Let's embark on this thrilling adventure together!
What is Generative AI?
Generative AI, or generative adversarial networks (GANs), is artificial intelligence involving two neural networks working together to generate new content. One network generates the content, such as images, music, or text, while the other evaluates and provides feedback. This process continues until the generated content is realistic and high-quality. Generative AI has various applications across different industries, such as creating realistic-looking photos of non-existent people or generating unique art pieces. It's an exciting technology with great potential for creativity and innovation.
Benefits of Using Generative AI
Here are some of the key benefits:
Creative Content Generation
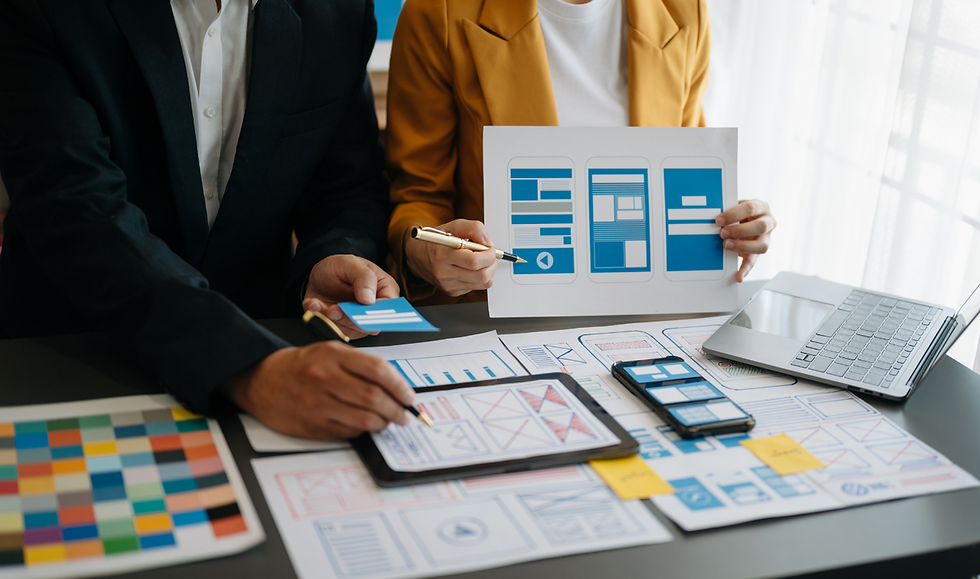
Generative AI's capacity for creative content generation marks a significant advancement in artificial intelligence. At the core of this capability lies the ability of generative models, such as Generative Adversarial Networks (GANs) and Variational Autoencoders (VAEs), to learn and replicate patterns inherent in various forms of data, including images, music, text, and video. Unlike traditional AI systems that rely on predefined rules or datasets, generative models can generate new content that exhibits characteristics similar to the training data.
In the context of creative industries such as art, design, and entertainment, this capability of generative AI opens up a world of possibilities. Artists and designers can leverage these models to explore novel concepts, experiment with unconventional ideas, and push the boundaries of traditional creative processes. For instance, artists may use generative AI to produce digital artworks that seamlessly blend different styles or to generate music compositions that evoke specific emotions.
Moreover, generative AI enables the democratization of creativity by providing accessible tools for individuals with varying artistic expertise. Platforms and applications powered by generative models empower users to engage in creative expression without requiring extensive technical skills or training. This democratization fosters a more inclusive, innovative community where diverse voices and perspectives can thrive.
Furthermore, generative AI enhances collaboration and co-creation in creative endeavors. Artists, designers, and other creative professionals can collaborate with generative models to co-author works, with the AI system serving as a creative partner or catalyst for creativity. This collaborative process accelerates the pace of creativity and leads to innovative outcomes that transcend individual contributions.
Data Augmentation
Generative AI's capability for data augmentation presents a valuable solution to one of the most persistent challenges in machine learning: the availability and quality of training data. Acquiring large and diverse datasets can be costly, time-consuming, or impractical in many domains. Moreover, datasets may suffer from imbalances, where certain classes or categories are underrepresented, leading to biased models.
Generative models, such as GANs and VAEs, offer a compelling solution to these data-related challenges by synthesizing new data samples that closely resemble accurate data. Machine learning models can generate artificial data points by learning the statistical properties of training data. These points can complement the original dataset, increasing its size and diversity.
The benefits of data augmentation extend beyond simply enlarging the dataset. Augmented data can help improve machine learning models' robustness and generalization capabilities. By revealing the model to a broader range of variations and scenarios, augmented data can help mitigate overfitting and improve the model's ability to generalize to unseen data.
Furthermore, data augmentation can address class imbalance issues by generating synthetic samples for underrepresented classes. This assists in preventing the model from being biased towards the majority classes and improves its performance on minority classes, leading to more equitable and accurate predictions.
In practical terms, data augmentation powered by generative AI has wide-ranging applications across various domains, including computer vision, natural language processing, and healthcare. For example, in medical imaging, where labeled datasets are often limited, and privacy concerns may restrict data sharing, generative models can generate synthetic medical images to augment the training data for disease diagnosis or anomaly detection tasks.
Personalization
Generative AI's ability to personalize content represents a significant advancement in marketing, e-commerce, and content recommendation systems, where consumers increasingly value tailored experiences. By leveraging generative models, businesses can create personalized content and recommendations that resonate with individual preferences and interests, ultimately enhancing customer engagement and satisfaction.
One critical advantage of generative AI in personalization is its capability to generate content dynamically adapted to each user's unique profile and behavior. Generative models can use user data like browsing history, purchase patterns, and demographics to produce personalized recommendations for products, services, or content highly relevant to each user.
Personalized content generated by generative AI can take various forms, including product recommendations, customized advertisements, or personalized user interfaces. For example, e-commerce platforms can use generative models to generate customized product recommendations based on a user's browsing and purchase history, increasing the likelihood of conversion and customer satisfaction.
Moreover, generative AI enables real-time adaptation and optimization of personalized content based on user interactions and feedback. As users engage with the customized content, their preferences and interests may evolve, necessitating continuous adaptation and refinement of the recommendations. Generative models can dynamically adjust the content generation process to reflect these changes, ensuring that the personalized experience remains relevant and engaging over time.
Personalization powered by generative AI benefits businesses by driving customer engagement and loyalty and enhances the overall user experience by providing content tailored to individual preferences and interests. Companies can utilize machine learning and neural networks to create personalized marketing, content delivery, and customer user experiences.
Prototyping and Design

Generative AI's role in prototyping and design signifies a transformative approach to creativity and innovation, particularly in architecture, product design, and fashion. At its core, generative models empower designers and creators to explore various possibilities by generating and iterating upon design variations based on specified constraints or objectives.
One key benefit of generative AI in prototyping and design is its ability to generate and evaluate numerous design alternatives rapidly. Traditional design processes often involve manual iteration and refinement, which can be time-consuming and labor-intensive. On the other hand, generative models automate this iterative process by quickly generating a diverse range of design options, allowing designers to explore and evaluate multiple concepts in a fraction of the time.
Moreover, generative AI enables designers to push the boundaries of creativity by generating unconventional and innovative design solutions. By leveraging machine learning techniques, generative models can identify patterns and relationships within design data, discovering novel design configurations and aesthetics that may not have been apparent through traditional design approaches.
Additionally, generative AI facilitates collaboration and co-creation in design projects by providing a platform for designers to interact with the generated designs and contribute their expertise and insights. Designers can use generative models for creativity, inspiration, and exploration, collaborating with the AI system to co-author designs that combine human creativity with machine intelligence.
Furthermore, generative AI supports sustainable design practices by optimizing designs for material usage, energy efficiency, and environmental impact. By simulating and analyzing the performance of generated designs under different conditions, designers can identify opportunities for improvement and optimization, leading to more sustainable and eco-friendly design solutions.
Anomaly Detection
Generative AI's application in anomaly detection signifies a powerful tool for identifying deviations or irregularities within datasets, offering crucial insights across various domains such as cybersecurity, fraud detection, and quality control. The fundamental principle underlying this capability lies in the ability of generative models to learn the underlying distribution of standard data and subsequently identify instances that deviate significantly from this learned pattern.
In cybersecurity, generative AI can be vital in detecting malicious activities or anomalies within network traffic, user behavior, or system logs. By training generative models on historical data representing normal network behavior, deviations indicative of potential security breaches or cyberattacks can be flagged for further investigation, helping organizations mitigate security risks and protect sensitive data.
Generative AI is useful in fraud detection as it recognizes unusual user behavior that may indicate fraudulent transactions or activities. By analyzing transactional data, user interactions, and other relevant features, generative models can identify anomalies indicative of fraudulent activity, enabling financial institutions and e-commerce platforms to detect and prevent fraudulent transactions in real time.
Moreover, generative AI supports quality control processes by detecting anomalies or defects in manufacturing, production, or supply chain operations. By analyzing sensor data, images, or other data collected during production, generative models can identify deviations from expected norms, alert operators to potential issues, and enable proactive intervention to maintain product quality and consistency.
Generative AI's ability to detect anomalies extends beyond identifying outliers in numerical data to detecting anomalies in complex data types such as images, text, and sequences. This versatility allows generative models to be applied to various applications. For instance, they can be used in medical diagnosis to identify anomalies in medical images or patient records. This can assist clinicians in the early detection and treatment of diseases.
Image Enhancement and Restoration
Generative AI's image enhancement and restoration capability represents a potent tool for enhancing the quality and fidelity of visual content across various applications and industries. At its core, generative models leverage sophisticated algorithms and neural network architectures to enhance images by restoring missing or degraded details, removing noise, and improving overall visual clarity.
Image super-resolution is a primary application of generative AI in image enhancement. It involves upscaling low-resolution images to higher resolutions while preserving key details and minimizing artifacts. By training on a dataset of high-resolution and corresponding low-resolution image pairs, generative models can learn to generate high-quality, high-resolution images from low-resolution inputs, effectively enhancing the visual fidelity of pictures captured at lower resolutions.
Generative AI also excels in image inpainting, which fills in missing or damaged regions within images. Whether removing unwanted objects from photographs or reconstructing missing parts of old or damaged photos, generative models can intelligently infer and generate plausible content to seamlessly blend with the surrounding areas, resulting in visually coherent and aesthetically pleasing reconstructions.
Moreover, generative AI offers solutions for denoising images by removing unwanted noise and artifacts while preserving important details and textures. Generative models can effectively reduce noise in photos by learning to differentiate between signal and noise in training data. This results in cleaner and more visually appealing visuals, particularly valuable in medical imaging, surveillance, and photography applications.
Style transfer is applying one image's visual style to another to create transformations. By separating content and style representations within images, generative models can generate new images that preserve the original's content while adopting another image's style characteristics, allowing for artistic expression and creative exploration.
Language Translation and Summarization
Generative AI's language translation and summarization prowess signifies a revolutionary advancement in natural language processing, offering transformative solutions for cross-lingual communication and information summarization. At its core, generative models leverage sophisticated neural network architectures and large-scale language datasets to learn complex patterns and structures inherent in language, enabling accurate translation between different languages and concise summarization of textual content.
Generative AI in language processing is commonly used for machine translation, automatically translating text from one language to another. Traditional machine translation approaches often rely on rule-based systems or statistical models, which may struggle to capture nuances and context-dependent meanings in language. Generative models, such as sequence-to-sequence models with attention mechanisms, capture these nuances by learning to generate translations based on contextual information and semantic similarities between languages.
Generative AI also offers solutions for text summarization, where lengthy documents or articles are condensed into shorter, more concise summaries while retaining important information and critical insights. By training on large text corpora and learning to identify salient information and relevant passages, generative models can generate summaries that capture the essence of the original text, facilitating efficient information retrieval and comprehension.
Moreover, generative AI enables multi-document summarization, where information from multiple sources is synthesized and condensed into a coherent summary. Whether it's summarizing news articles, research papers, or social media posts, generative models can ingest and analyze diverse sources of information to generate comprehensive summaries that capture the essential points and perspectives across different texts.
Generative models can also be applied to paraphrasing tasks, where text is rephrased or reworded while preserving the original meaning and intent. By learning to generate paraphrases through training on paired sentences with similar meanings, generative models can facilitate better understanding and interpretation of text by providing alternative formulations and expressions.
Medical Imaging and Diagnosis
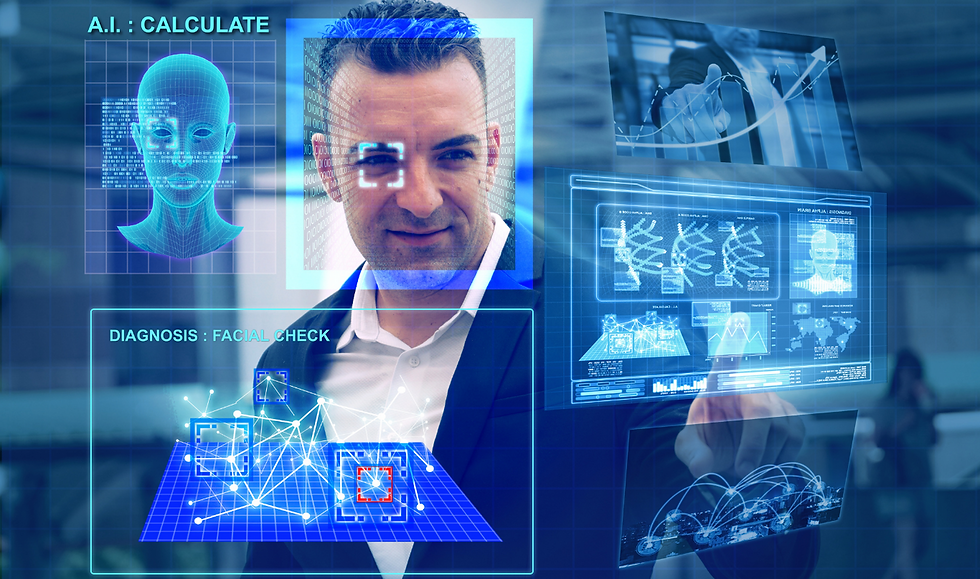
Generative AI's application in medical imaging and diagnosis represents a groundbreaking advancement with profound implications for healthcare. Generative models, powered by deep learning and neural networks, offer medical professionals more accurate diagnosis, treatment planning, and research by facilitating transformative solutions for medical image synthesis, reconstruction, and analysis.
One of the essential applications of generative AI in medical imaging is image synthesis, where realistic synthetic medical images are generated to augment limited or unavailable datasets. In scenarios where labeled medical images are scarce or challenging to obtain due to privacy concerns or rare medical conditions, generative models can generate synthetic images that closely resemble accurate patient data, enabling more robust training of machine learning algorithms for disease classification, segmentation, and detection.
Generative models also excel in image reconstruction, where noisy or low-quality medical images are enhanced to improve diagnostic accuracy and visual clarity. Generative models can effectively denoise images, enhance contrast, and improve spatial resolution by learning to differentiate between signal and noise in medical images. This results in more transparent and more interpretable images for clinicians and radiologists.
Moreover, generative AI offers solutions for image-to-image translation, where medical images captured using different modalities (e.g., MRI, CT, PET) are converted into a standard representation for easier comparison and analysis. By learning to map images between different domains, generative models enable seamless integration of multimodal imaging data, facilitating comprehensive evaluation and diagnosis of medical conditions.
Generative models can also assist in data augmentation for medical imaging datasets, generating synthetic variations of existing images to increase dataset size and diversity. By generating variations such as different anatomical views, pathology types, or imaging artifacts, generative models can enhance the robustness and generalization capabilities of machine learning algorithms trained on medical image data, leading to more reliable and accurate diagnostic models.
Game Design and Virtual Worlds
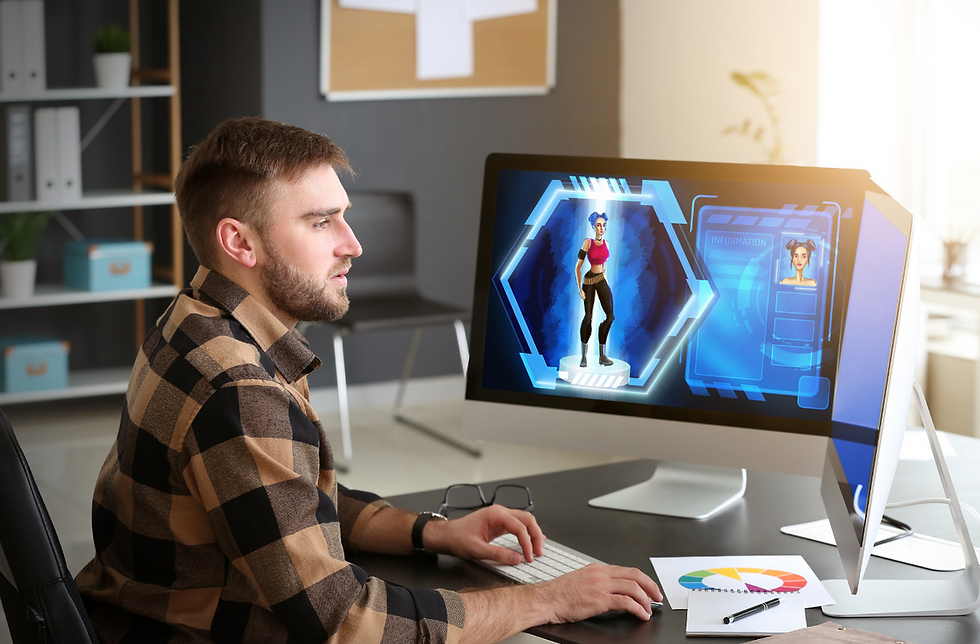
Generative AI's application in game design and virtual worlds presents a paradigm shift in developing interactive experiences, offering innovative solutions for creating dynamic and immersive virtual environments. Leveraging sophisticated machine learning algorithms and neural network architectures, generative models empower game developers to generate content, design levels, and create virtual worlds that adapt and respond to player actions and preferences in real-time.
Procedural content generation is a primary use case of generative AI in game design, where game assets like landscapes, terrain, and objects are algorithmically generated rather than crafted manually. By training generative models on vast datasets of existing game assets, developers can create procedural generation algorithms that produce diverse and realistic environments, enhancing the replayability and variability of games.
Generative models also excel in level design, where the layout and structure of game levels are generated automatically based on predefined design principles or player preferences. By learning from player interactions and feedback, generative models can dynamically adjust the difficulty level, pacing, and content to provide each player with a tailored and engaging experience, fostering immersion and enjoyment.
Moreover, generative AI offers content adaptation and personalization solutions in virtual worlds, where game environments and narratives are dynamically customized to reflect individual player characteristics, preferences, and progression. By analyzing player behavior and interaction patterns, generative models can generate personalized content such as quests, challenges, and storylines that align with each player's play style and objectives, enhancing player engagement and satisfaction.
Generative models can also be applied to procedural animation, where character movements and behaviors are generated algorithmically to create lifelike and dynamic animations. By learning from motion capture data and biomechanical principles, generative models can develop realistic and responsive animations that adapt to changing game conditions and player inputs, enhancing the realism and immersion of virtual characters and creatures.
Assistive Tools for Creativity
Generative AI's role as an assistive tool for creativity represents a significant advancement in supporting and augmenting human creative endeavors. Generative models, which use machine learning algorithms and neural networks, assist creatives with idea generation, inspiration, and automation of repetitive tasks.
Generative AI is increasingly used to help artists and designers generate and explore new concepts and designs. By analyzing vast datasets of existing artworks, generative models can generate variations, combinations, and reinterpretations of artistic styles, motifs, and themes, providing artists with fresh perspectives and inspiration for their creative endeavors.
Generative models also serve as valuable tools for brainstorming and idea generation in creative projects. By providing prompts, suggestions, and variations based on user input, generative AI can stimulate creative thinking and spark new ideas, helping individuals overcome creative blocks and generate innovative solutions to artistic and design challenges.
Moreover, generative AI enables the automation of repetitive tasks and workflows in creative processes, freeing up time and cognitive resources for artists and designers to focus on more complex and expressive tasks. Whether it's automating repetitive tasks such as image retouching, layout design, or pattern generation, generative models streamline workflows and enhance productivity in creative projects.
Generative models can also be applied to collaborative creative processes, facilitating co-creation and exploration of ideas among multiple individuals. By providing a platform for shared creativity and experimentation, generative AI fosters collaboration and cross-pollination of ideas, leading to the emergence of novel concepts and creative expressions that transcend individual contributions.
Limitations of Using Generative AI
While generative AI holds immense promise and has demonstrated remarkable capabilities across various domains, it also has several limitations and challenges that must be addressed.
Here are some fundamental limitations of using generative AI:
Data Dependency: Generative models require high-quality training data to learn meaningful representations and generate realistic outputs. Limited or biased training data can direct to poor performance and generalization of generative models, limiting their applicability in real-world scenarios.
Mode Collapse: Generative Adversarial Networks (GANs) are a widely used type of generative model. However, they are susceptible to a problem known as mode collapse. This means that the model may not capture the full range of diversity in the training data and instead produces repetitive or unrealistic outputs. Overcoming mode collapse is still a challenging issue in GAN training.
Evaluation Metrics: Assessing the quality and fidelity of generated outputs remains a subjective and challenging task. Traditional evaluation metrics may not accurately capture the nuances of generated content, making it difficult to quantify the performance of generative models objectively.
Robustness and Security: Adversarial attacks can significantly alter the generated outputs of generative models through small perturbations in input data.Ensuring the robustness and security of generative models against such attacks is an ongoing research challenge.
Ethical and Legal Implications: Generative AI raises ethical concerns about privacy, consent, and the potential misuse of generated content for malicious purposes, including deepfakes and misinformation. Addressing these moral and legal implications requires careful consideration and regulation.
Computational Resources: Training and deploying generative models often require significant computational resources, including high-performance hardware and large-scale infrastructure. The computational complexity of generative AI can pose challenges for researchers and practitioners with limited resources.
Interpretability: Understanding and interpreting the internal workings of generative models can be challenging, especially for complex neural network architectures. Lack of interpretability hinders trust and transparency in decision-making, particularly in critical applications such as healthcare and finance.
Bias and Fairness: Biases in training data for generative models can lead to unfair outcomes. Ensuring fairness and mitigating biases in generative AI systems requires careful attention to data selection, model design, and evaluation strategies.
Addressing these limitations requires interdisciplinary collaboration and ongoing research efforts to develop robust, reliable, and ethical generative AI systems that meet real-world applications' diverse needs and challenges.
7 Best Generative AI Tools
When to Use Generative AI
Here are some instances when using generative AI is beneficial:
Creating Marketing Content
Generative AI is a potent tool for generating marketing content across various mediums, such as text, images, videos, and audio. Whether you need blog posts, photo captions, social media copy, or product descriptions, AI can assist in content creation, helping you save time and effort. Additionally, generative AI can produce photorealistic art and graphics to enhance your marketing campaigns. However, it's essential to have a human editor review AI-generated content to guarantee it aligns with your brand's voice, maintains factual accuracy, and prevents bias.
Customer Service and Technical Support Chatbots
Generative AI can be deployed to create customer support chatbots, streamlining response times to customer inquiries and freeing up human representatives to handle more complex issues. Whether integrated into your website or SMS support system, AI-powered chatbots can immediately assist customers, enhancing overall satisfaction and efficiency in customer service operations.
Writing Email Responses
Sales representatives can leverage generative AI to compose personalized email responses quickly and efficiently. By automating the process of drafting messages, AI can generate custom emails tailored to specific tones and contexts, saving time for sales teams and ensuring consistency in communication. Additionally, AI can integrate with CRM systems to extract relevant data and personalize messages further, enhancing customer engagement and relationship management.
Product Demonstrations
Generative AI is valuable for creating product demonstrations, particularly in generating demo videos or providing suggestions for improving user experience. AI tools can assist in scripting demo videos, answering queries during post-production, and even generating synthetic customer data for anonymized product demonstrations. By harnessing generative AI, businesses can enhance the effectiveness and realism of product demonstrations while streamlining the production process.
How to Use Generative AI
Generative AI involves several steps, from selecting an appropriate model to training it on relevant data and deploying it for specific tasks.
Here's a general guide on how to use generative AI effectively:
Select a Generative Model: When selecting a generative model, consider the type of data, the complexity of the task, and your available computational resources.
Prepare Training Data: Gather and preprocess a large and diverse dataset that reflects the domain and characteristics of the task you want to tackle. Ensure the data is clean, well-annotated, and representative of the target distribution.
Train the Model: Train the generative model on the prepared dataset using appropriate training algorithms and techniques. This typically involves feeding the model with input data and optimizing its parameters to minimize a predefined loss function, such as reconstruction error or adversarial loss.
Fine-tune the Model (Optional): Fine-tune the trained model on specific tasks or domains to improve its performance or adapt it to new data distributions. Fine-tuning involves further training the model on task-specific datasets or adjusting its hyperparameters.
Generate Output: Once the model is trained or fine-tuned, you can generate output samples relevant to your task. Depending on the model and application, this could involve generating images, text, music, or other types of content based on specified input or constraints.
Evaluate Output Quality: Assess the quality and fidelity of the generated output using appropriate evaluation metrics and human judgment. This step is crucial for ensuring that the generated content meets the desired criteria and is suitable for the intended application.
Iterate and Refine: Iterate on the model training, fine-tuning, and evaluation process to improve performance and address shortcomings or issues. This may involve experimenting with different architectures, hyperparameters, or training strategies.
Deploy the Model: Deploy the trained generative model for specific tasks or applications, such as content generation, recommendation systems, or creative tools. Ensure that the deployment environment meets the model's computational and scalability requirements.
Monitor Performance: Continuously monitor the deployed model's performance in real-world scenarios and collect feedback from users or stakeholders. This allows you to identify performance degradation or issues and take corrective actions.
Update and Maintain: Periodically update and maintain the generative model to incorporate new data, adapt to changing requirements, and address emerging challenges. This may involve retraining the model with updated datasets or fine-tuning its parameters based on evolving needs.
By following these steps and best practices, you can effectively harness the power of generative AI to develop, innovate, and solve complex problems across various domains.
The Bottom Line
In conclusion, generative AI holds incredible potential to revolutionize various industries and creative fields. This comprehensive guide has explored the applications, techniques, and ethical considerations surrounding generative AI. As we continue to harness this technology, it's essential to prioritize ethical and responsible use while embracing the boundless opportunities for innovation and creativity. By following the guidelines in this manual, we can confidently navigate generative AI's evolving landscape.
Comments